Profusion is now part of Atombit!
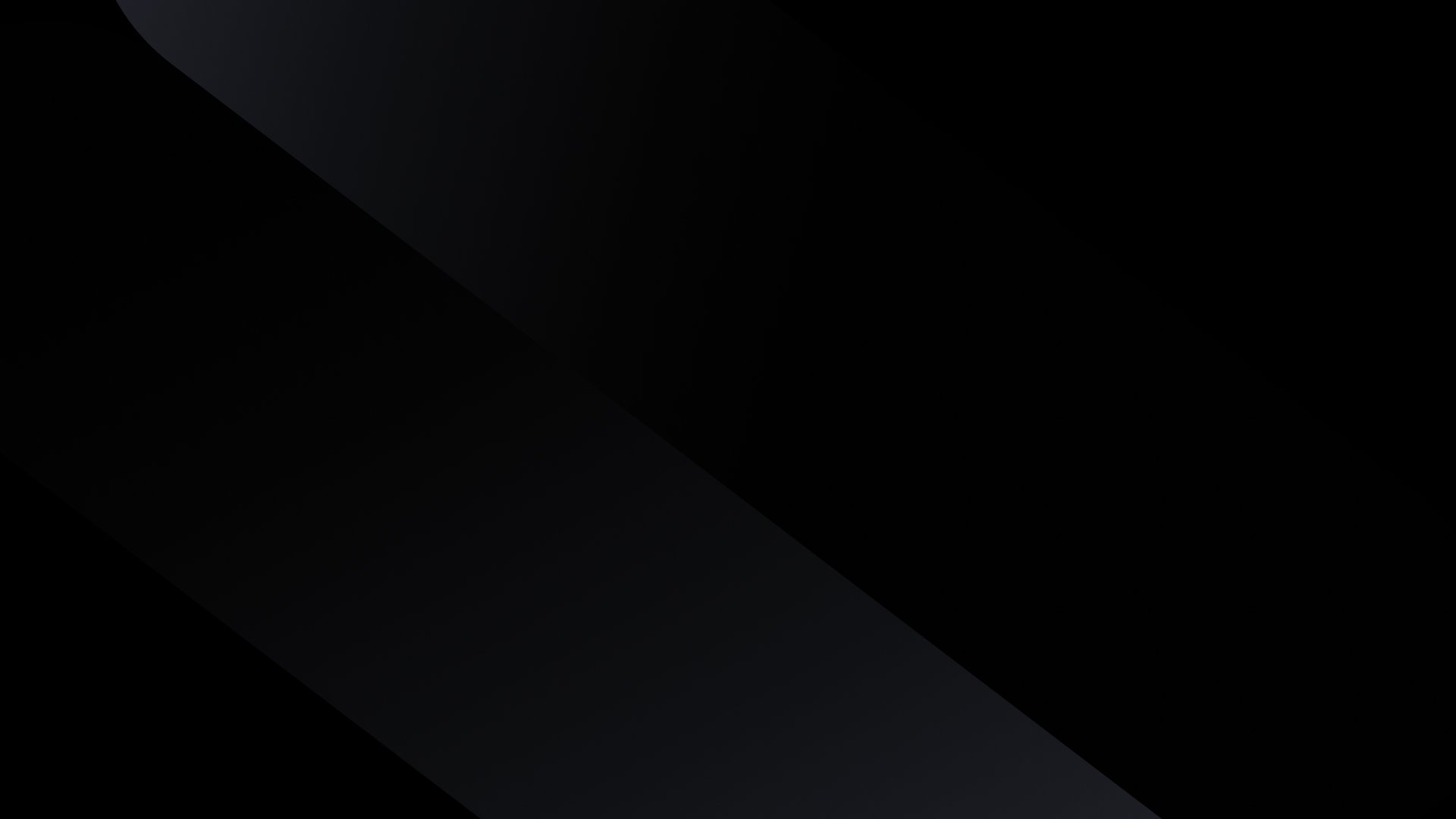
Powering business growth with world-class Data, AI & Adaptive CRM Services
We are experts in giving businesses of any size the tools they need to get the most out of AI and their data assets.
Data and AI
We provide the complete package from platform migration and strategy through to campaign management and execution.
Adaptive CRM
Data and AI
We are experts in giving businesses of any size the tools they need to get the most out of AI and their data assets. Everything from building data infrastructure through to designing and deploying AI solutions can be executed by our team of data engineers, software developers, analysts, scientists and cyber security experts.
Adaptive CRM
We have blended best-in-class digital marketing and data science techniques to create a CRM strategy that evolves alongside your business, ensuring you are always equipped to meet the ever-changing needs of your customers and the market.
We provide the complete package from platform migration and strategy through to campaign management and execution.